Estimating Economic Efficiency Levels and Identifying Its Determinants for Milk Producers’ Households in North Shewa Zone, Oromia Region, Ethiopia
Gadisa Girma1*, Nigusu Abera 2, Yimenu Kassa3, Zewditu Berhun4, Abi Tolosa5, Genet Shiferaw6
1 Department of Agricultural Ecoomics, Salale University, Ethiopia (Email: gadisag2@gmail.com; Phone: +251924031078)
2Department of Agricultural Economics, Salale University, Ethiopia (Email: boonaaifaa@gmail.com), 3 Department of Agricultural Economics, Salale University, Ethiopia (Email: baymfbt@gmail.com) 4Department of Agribusiness and Value Chain Management, Salale University, Ethiopia (Email: zewditube23@gmail.com)5 Department of Animal Science, Salale University, Ethiopia (Email: abitolossa6@gmail.com) 6 Department of Agricultural Economics, Salale University, Ethiopia (Email: iyaya2007@gmail.com)
ARTICLE INFORMATION | ABSTRACT |
*Corresponding author: Gadisa Girma E-mail: gadisag2@gmail.com Keywords: Efficiency Ethiopia Milk Stochastic Frontier Two-limit Tobit | This study aimed to estimate economic efficiency levels and identify its determinants for milk producers’ households in North Shewa Zone, Oromia Region, Ethiopia. Three stages random sampling technique was used to select 400 sample farmers. The data were analyzed using descriptive statistics and an econometrics model. The result of the stochastic frontier model showed significant and positive elasticity of lactation cow, green forage, and crop residue. The estimated mean values of technical, allocative, and economic efficiency were 58%, 77.6%, and 44.7% respectively. The yield gap due to technical inefficiency was 9.6 liters per cow per day. A two-limit Tobit model result shows that education, amount of concentrate feed used, grazing land, type of breed, and frequency of extension contact contributed significantly and positively to technical efficiency. Moreover, total land, dairy farm experience, dairy membership, and type of breed affect allocative efficiency significantly and positively while the amount of concentrate feed used had a significant and negative effect on allocative efficiency. Economic efficiency is also affected significantly and positively by education level, total land, grazing land, type of breed, and frequency of extension contact. To improve the efficiency level of farmers, due attention should be given to the use of concentrate feed, improving feed availability, adequate and proper management of grazing land, and using of improved breed and dairy cooperatives. |
ARTICLE INFORMATION ABSTRACT
Ethiopia has the tenth-largest livestock inventory in the World. The country has the largest number of livestock, more than any other country in Africa. Ethiopia leads with a staggering 60.39 million cattle, while Tanzania, in the second position, has an estimated total of 33.9 million cattle (Africa Census, 2020). Though Ethiopia has a large livestock inventory, the productivity of cattle remains low.
According to Central Statistical Agency (CSA) (2020), there are around 7.56 million dairy cows in Ethiopia. Of these, 15.04 million are milking cows. On average, each cow produces 1.48 liters of milk daily. Nathaniel et al. (2014) indicated that dairy inputs and service provisions are still at the infant stage and the development of improved dairy cows is limited in the country.
The increase in milk production may have come mostly from the increased number of cows rather than increased productivity. Nega and Simeon (2006) indicated the inefficiency among smallholder dairy producers due to the inefficient use of scarce resources. Understanding the existence of inefficiency and different factors contributing to the inefficiency by farmers and policymakers helps to improve efficiency with a view to bringing a desired change in the sector. However, most efficiency studies in agricultural economics focus on technical efficiency, which is just one component of overall economic efficiency.
Focusing only on technical efficiency (TE) understates the benefits that producers could from improvements in overall performance. Unlike technical efficiency, research done on economic efficiency, especially in milk production is limited. In addition, many empirical studies did not consider yield gaps because of technical inefficiency among milk producers.
North Shewa Zone, Oromia Region in Ethiopia has milk production potential, and the demand for milk and milk products has been increasing while output is not able to meet the higher demand. Moreover, there is an output difference among dairy producers.
Dairy producers have little knowledge of how to use minimum cost (cost efficiency) in the study area. Therefore, knowledge about the level of economic efficiency of smallholder milk production and the underlying socio-economic and institutional factors causing inefficiency may help to assess the opportunities for increasing milk production. Additionally, to the best of knowledge, no studies have been conducted in the area of economic efficiency (EE) of milk production, especially in the study area.
Hence, there is a need to fill the existing knowledge gap by addressing issues related to technical, allocative efficiency (AE), and EE of smallholder milk production in the study area by providing empirical evidence on smallholder milk producers. Therefore, the objective of this study was to estimate economic efficiency levels and identify the determinants for milk producer households in North Shewa Zone, Oromia Region, Ethiopia.
METHOD AND MATERIALS
Study Area
This study was conducted in the North Shewa Zone of Oromia Regional state, Ethiopia due to its high potential
in milk production. It has a total of 13 districts and is bordered on the South by Oromia Special Zone Surrounding Addis Ababa, on the South West by West Shewa, on the North by the Amhara Region, and on the South East by East Shewa.
Sampling Techniques and Sample Size Determination
Three stages of random sampling procedures were employed to draw a representative sample. In the first stage, four districts, Degem, Wuchale, Debra Libanos, and Girar Jarso, out of 13 milk producing districts in the zone, were purposively selected.
In the second stage, two kebeles from each district, with a total of eight kebeles from four sampled districts, were selected purposively due to their high dairy production potential. In the third stage, 400 sample farmers were selected using a simple random sampling technique based on probability proportional to the size of milk producers in each of the eight selected kebeles. The sample size was determined by using the formula provided by Yamane (1967).
Accordingly, the sample size for the study is determined based on the following formula:
Where, n = sample size (including the non-response rate of 1%), N = Total milk producers in the study area, and e = Level of precision considered.
Table 1: Sample size distribution
No. | Name ofsampleddistrict | Totalhousehold milkproducers | Sampledhousehold | Proportion (%) |
1 | Degem | 5570 | 60 | 15.00 |
2 | Wuchale | 13880 | 149 | 37.25 |
3 | Debralibanos | 4273 | 46 | 11.50 |
4 | Girarjarso | 13520 | 145 | 36.25 |
Total | 37243 | 400 | 100 |
Source: North Shewa Livestock and Fishery Development Office (2020)
Types, Sources, and Methods of Data Collection
The research is accomplished using primary and secondary data sources, which are qualitative and quantitative in nature. The primary data necessary to achieve the designed objectives were obtained from sample households through a structured questionnaire for sampled households and a checklist for focus group discussion and key informants interviews. Secondary data was collected from relevant sources such as articles, proceedings, journals, CSA, and district annual reports which were vital to the study.
Data measurement
i. Output variable: It is defined as the actual quantity of milk produced and measured in liters (L) during the 2020 production year by sample households. This is a dependent variable of the production function taken as a continuous variable.
ii. Input variables: Defined as the total inputs used by sample household in the production of milk namely: lactation cow (number), labor (Man-day), Green forage (beli), and crop residue (beli) in the 2020 production year (1beli=1kg).
iii. Dependent variables: The dependent variables for this study are; TE, AE, and EE scores of milk production obtained from the stochastic frontier function.
iv. Inefficiency variables
1. Sex: This is a dummy variable that was measured as 1 if the household head is male and 0, otherwise.
2. Education: It is a continuous variable that is defined as the education level of the sample household head. This variable was measured in terms of years of schooling.
3. Concentrate: the total amount of concentrate used by sampled households to produce milk in quintals (Qt).
4. Total land: refers to the total area cultivated (owned, shared, or rented in) land that the sample household managed during the 2020 production year measured by a hectare (ha).
5. Extension: The frequency of extension agents contacting farmers and vice versa, measured by the number of contact per production year.
6. Grazing land: it refers to the total grazing land area allotted by the sample household for cow milk production during 2020 that was measured in ha.
7. Type of breed: It is a categorical variable that takes a value of 1 if the farmers use local breed, 2 if the farmers use both local breed and cross-breed, and 3 if the farmers use cross-breeding cows.
8. Dairy experience: It is a continuous variable and refers to the total years that the household participated in milk production, which is measured in years.
9. Distance: It is defined as the distance of the nearest market from the house of the household head in walking minutes.
10. Membership: It is the dummy variable that takes a value of 1 if the sampled farmer is in a dairy cooperative member and 0 otherwise.
11. Feeding method: It is a dummy variable equal to 1 for the farm that uses the total mixed ratio (TMR) and 0 if the farm uses the pasture feeding method.
12. Housing System: It is a dummy variable that takes 1 for farms that use free stall housing and 0 otherwise.
Method of Data Analysis Descriptive statistics
Descriptive statistics such as mean, minimum, maximum, percentages, frequencies, and standard deviation or standard error were applied to describe demographic, socio-economic, farm characteristics, institutional characteristics, and distribution of efficiency levels of milk producers in the study area. After coding and feeding the collected data into the computer, STATA version 15 was used for the analysis.
Econometric analysis
Specification of an econometric model
Coelli et al. (1998) recommended that the Stochastic Frontier Production Function (SFPF) is more appropriate than DEA and deterministic models in agricultural applications, especially in developing countries, where measurement errors generally influence the data are generally influenced by measurement errors and the effect of weather, disease, and pests play a significant role.
Some researcher argues that Cobb-Douglas functional form has advantages over the other functional forms in that it provides a comparison between the adequate fit of the data and computational feasibility. It is also convenient in interpreting the elasticity of production and it is very parsimonious with respect to degrees of freedom and it is convenient in interpreting elasticity of production.
In addition, the Cobb-Douglas production function is attractive due to its simplicity and because of the logarithmic nature of the production function that makes econometric estimation of the parameters a simple matter.
The translog production function is more complicated to estimate the parameters having serious estimation problems. One of the estimation problems is as the number of variable inputs increases, the number of parameters to be estimated increases rapidly. Another problem is the additional terms require cross-products of input variables, thus making a serious multicollinearity problem (Coelli, 1995). Therefore, this study used stochastic production frontier to estimate the TE, AE, and EE levels of smallholdermilk-producing farmers in the study area.
Following Aigner et al. (1977) and Meeusen and Van den Broeck (1977), the general functional form of the stochastic frontier model for this study is specified as follows:
Where z = 1, 2, 3… n; Yz represent the observed milk output level of the zth sample farmer; f (Xz; βz) is the convenient frontier production function (e.g. Cobb
Douglas or Trans log); Xz denotes the actual input vector by the zth farmer; βz stands for the vector of unknown
parameters to be estimated; ɛz is a composed disturbance term made up of two error elements (Vz and Uz) and n represents the number of farmers who will involve in the survey.
The stochastic frontier functional approach requires a priori specification of the production function to estimate the level of efficiency. Among the possible algebraic forms, Cobb-Douglas and trans-log functions were the most popularly used models in the most empirical studies of agricultural production analysis. Therefore, the Cobb Douglas production function was adopted for this study. Thus, the Cobb-Douglas frontier function was specified as follows:
The linear form of Cobb-Douglas production functions for this study was defined as:
Where, ln denotes the natural logarithm (i.e., base e); j represents the number of inputs used; z represents the zth farm in the sample; Yz represents the observed milk output of the zth sample farmer; Xjz denotes zth farm input variables used in milk production of the zth farmer; β_0 represent intercept; β_1-β_4 stands for the vector of unknown parameters to be estimated and represent elasticity of milk production; Ɛz is a composed disturbance term made up of two error elements (Vz and Uz); the symmetric component (Vz) is assumed to be independently and identically distributed as random errors with zero mean and variance N (0, σ2v), which captures inefficiency as a result of factors beyond the control of farmers and Uz proposed to capture inefficiency effects in the production of milk.
Assuming that the production function in equation (4) is self-dual. Cobb Douglas), the dual cost function of the Cobb-Douglas production function can be specified as:
Where z refers to the zth sample farm; j is the number of inputs; Cz is the minimum cost of production; Wjz denotes input prices of z th farm; Y* refers to milk output in litre; α’s are parameters estimated; Vz denotes random variables assumed to be independent and identically distributed random errors with zero mean and variance and Uz denotes non-negative random variables which are assumed to account for cost inefficiency and assumed to be independent and identically distributed random errors with zero mean and variance.
Sharma et al. (1999) suggest that the corresponding dual cost frontier of the Cobb-Douglas production functional form in equation (5) can be rewritten as:
The economically efficient input vector of the zth farm Xze is derived by applying Arega and Rashid (2005) and substituting the firms input prices and adjusted output level, a system of minimum cost input demand equation can be expressed as:
We can define the farm-specific TE in terms of observed milk output (Yz) to the corresponding frontier milk output (Y*) using the existing technology.
The cost efficiency of an individual farm is defined in terms of the ratio of the observed cost (C) to the corresponding minimum cost(C*) given the available technology. That is, cost efficiency (CE):
Where the observed cost (C) represents the actual production cost whereas the minimum (efficient) cost (C^* ) represents the frontier total production cost or the least total production cost level.

The farm-specific AE is defined as the ratio of minimum total production cost (C*) to the actual observed total production cost (C). Following Ali et al. (2012), the EE index was derived from equations (8) and (9) as follows:

Determinants of inefficiencies

Where: , a latent variable representing the efficiency scores of farm z (TE, AE and EE); intercept; β_kunknown parameter; Xkz are demographic, institutional, socio-economic and farm-related variables which are expected to affect TE, AE and EE; k is a number of explanatory variables that affect TE, AE and EE and Uz are an error term that is independently and normally distributed with mean zero and variance σ2.
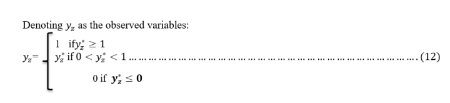
Likelihood ratio statistic
Aigner et al. (1977) proposed the log likelihood function for the model in equation (3) assuming normal distribution for the technical inefficiency effects (Uz). They expressed the likelihood function using λ parameterization, where λ is the ratio of the standard errors of the non-symmetric to symmetric error term (i.e. λ= σ U/ σ v). According to Bravo and Pinheiro (1997) gamma (γ) can beformulated as:

In this study, the likelihood ratio test was conducted to select the appropriate functional form that best fits the data. The value of the generalized likelihood ratio (LR) statistic to test the hypotheses that all interaction terms, including the square specification, is equal to zero (H0: βjz=0) was calculated as follows.
Following Greene (2003) the hypothesis tests were conducted using the log-likelihood ratio (LR) statistics, λ which is defined in equation (14):

Where: LR= Generalized log-likelihood ratio L (Ho) = Denotes the likelihood function value under the null (Ho) L (H1) = Denotes the likelihood function value under the alternative hypothesis (H1)
This value was compared with the upper 5% point for the χ^2distribution and the decision was made based up on the model result. If the calculated χ^2 value is less than the tabulated upper 5 percent point of the critical value, we accept the specified null hypothesisis at a 5 percent level of significance.
Milk yield gap
Yield gap is the difference between yield potential and actual farmers’ yields over a given spatial or temporal scale (Ittersum et al. 2013). The study measured the milk yield gap to determine how much milk output is lost because of inefficiency variation among milk-producing farmers in the study area. From the stochastic model defined in equation (15), TE of the zth farmer was estimated as follows.

Then solving for , Yz the potential milk output (liter/cow/day) of each sample household is represented as:

TEz= technical efficiency of the zth sample household in milk production the frontier or potential output of the zth sample household in milk production in liter/cow Yz=the actual or observed output of the zth sample household farmer in milk production in liter. Hence, milk yield gap (liter/cow/day) =potential yield (liter/cow/day)- actual yield (liter/cow/day).
Thus, Milk Yield gap = – Yz…………………………………………………………………………(16)
RESULTS AND DISCUSSIONS
Descriptive Statistical Results
Table 2 below shows that about 10.75% of the sample households were female-headed and the remaining 89.25% were male-headed. It was understood that female-headed households in rural areas in Ethiopia face more challenges in dairy production and marketing compared with their male-headed counterparts.
This is partly due to cultural barriers and their busy schedules as they are engaged in domestic, reproductive, and community roles. Moreover, from the total sampled household, 2.5%, 50%, and 47.5% are using local, both local and cross breed and cross breed milking cows in the study area respectively. This indicates that the majority of the sampled household use both crossbreed and local as well as crossbreed only.
Table 2 also illustrates that 69.5% of the sampled household use free stalling while 30.5% do not use free stalling. The result shows that most of the sampled farmers use free stalling in which cows are “free” to move around to eat, drink and rest wherever they like. These barns provide easy access to feed and clean water, as well as shade and protection from inclement weather which in turn increase the productivity of the milking cow.
The feeding method is important to improve the productivity of the milking cows there by the associated efficiency would increase than pasture feeding method. The study shows that from the sampled household, 76% use total mixed ratio while the rest 24% not use. The finding implies that most of the sampled milk producers use total mixed ratio in the study area. Related with dairy membership, around 75.25% of the sampled households are not participating in dairy member while 24.75% are participating in dairy cooperative member.
This indicates that the majority of the sampled household in the study area are not participate in dairy membership. Farmer who participates in dairy cooperative can get different information, training, market access and etc. this leads them to become more efficient than who do not participate in dairy cooperative member.
Table 2: Descriptive statistics of dummy variables
Variables | Description | Frequency | Percent |
Sex | Male | 357 | 89.25 |
Female | 43 | 10.75 | |
Type of breed | Local | 10 | 2.5 |
Both | 200 | 50 | |
Cross | 190 | 47.5 | |
Housing system | Not | 122 | 30.5 |
Free stall | 278 | 69.5 | |
Feeding method | Not | 96 | 24 |
Total mixed ratio | 304 | 76 | |
Dairy membership | Not member | 301 | 75.25 |
Member | 99 | 24.75 |
Source: Own computation (2020)
In Table 3 below the descriptive statistics of total land, grazing land, amount of concentrate feed used, frequency of extension contact, distance of the from home to the nearest market and Total livestock owned were discussed.
Land is the main resource needed by the milk producers to earn their livelihoods. Farmers use most of their land for crop production. The average total land of the sampled milk producers’ was about 2.24 ha (Table 3). The result implies that households in the study area have relatively larger land size compared to that of the national average of farmers in Ethiopia which is 1.2 ha. If total land increases, dairy cows gets more outputs from crop production (stover of sorghum, teff, wheat, fababean, and etc) to feed their cows.
Grazing land is the main resource needed by the farmers to feed their livestock (like milking cow) which is the main source of feed by providing different fodder, grasses and etc. The average total grazing land of the sampled milk producers was about 0.48 ha with a minimum of 0 ha and 3ha (Table 3). Farmer who has large grazing land has the opportunity to get high yield of milk than the others in the study area since grazing land provides feed for lactation cows.
Concentrate is one of the types of feed used in most of milk producers in the study area which is used to increase the production and productivity of lactation cow. The study indicates that, on average, the sampled farmers use 20.58qt of concentrate feed for cows per lactation period with a minimum of 0 (not used) and maximum of 1300qt (Table 3). This implies that most dairy farmers in the study area use concentrate feed for their milking cows as feed to get more milk productivity per day and per lactation period.
Extension work in the study area focuses on the provision of general advisory services on major dairy production practices (such as proper feeding system, housing system, veterinary services on timely and how farmers manage their milking cows day to day), and also give how the farmers become dairy cooperative member to get different information especially on the price of milk.
Development agents have been giving extension services in their respective field of specializations. They are required to advice and follow up their farmer’s dairy farm. The survey result also indicated that frequency of extension contact in 2019/20 production year(lactation period) was on average about 4.34 with the maximum contact of like 24 times and minimum 0 times (no contact) times per lactation period (Table 3).
Distance is the time span required to reach the nearest market from homestead of the milk producers farmers and is essential variable in explaining the capacity of the farmers’ performance. And it refers to how long time it takes (in walking minutes) for a dairy farmers to sell their milk and buy different inputs such as concentrate feed. It is an important variable due to the fact that as the farmers’ home located far from nearest market, there would be limited access to get inputs easily and on timely which is very important in dairy production.
Moreover farmers whose house is near to the market can easily get information on price of milk and provide also their product to the market in a short period of time. The study illustrate that distance from home to the nearest market in man walking minute was on average 45.84 with the maximum 180 minutes and minimum 1 minutes in the study area (Table 3).
Given a mixed farming system in the study area, livestock has imperative contribution for household income and food security. This income is very important especially to buy feeds for milking cows. The type of livestock kept by sampled farmers includes cow, oxen, bull, horse, mule, donkey, calf, goat, and heifer.
Among others, oxen power is the major input in crop production process serving as a source of draft power which at the end produce different crop by products that is used as fodder for milking cows. On average, the livestock holding of the sampled farmers in the study area was 4.69TLU per household with a minimum of 0 (no livestock other than milking cows) and a maximum of 17.77 in TLU (Table 3).
Table 3: Descriptive statistics of continuous variables
Variable description | Mean | Std.Dev. | Minimum | Maximum |
Family size(AE) | 3.86 | 1.63 | 1 | 9.05 |
Education (year of schooling) | 3.69 | 3.84 | 0 | 15 |
Dairy farm experience(years) | 14.96 | 10.73 | 1 | 60 |
Total land(ha) | 2.24 | 1.72 | 0.125 | 10 |
Grazing land(ha) | 0.48 | 0.46 | 0 | 3 |
Amount of concentrate feed used(qt) | 20.58 | 77.68 | 0 | 1300 |
Frequency of Extension(number) | 4.34 | 14.29 | 0 | 24 |
Distance from home to market(minute) | 45.84 | 31.75 | 1 | 180 |
Total livestock owned (TLU) | 4.69 | 2.67 | 0 | 17.77 |
Source: Own computation (2020)
Inputs used for milk production and cost function
The production function for this study was estimated using four input variables. On average, sample households produced 4989.03 lit of milk per lactation period, which is the dependent variable in the production function. The number of lactation cows, by sample households during the study, ranged from 1 to 9 with an average number of 2.94.
On average, the amount of human labour, green forage, and crop residue used by the sampled milk producers was 717.45 man day (MD), 202.3 qt, and 38.2 qt respectively(qt=quintals). Among the various cost factors of production, the cost of lactation cow accounted for the highest share (56112.5 birr).
Following the cost of lactation cow, the cost of labor takes a major share out of the total cost of production which is 21523 birr. Besides, the cost of crop residue takes the smallest share (3152.56 birr) out of the total cost of milk production (Table 4).
Econometric Results
Hypothesis Testing
The first null hypothesis tested was test for the selection of the appropriate functional form for the data; Cobb Douglas versus Translog production function. The functional form that can best fit the data was selected by testing the null hypothesis. The result indicated that the null hypothesis was accepted and Cobb-Douglas functional form best fits the data. The second null hypothesis tested was the test for the existence of the inefficiency component of the composed error term of the Stochastic Frontier Model (SFM).
This is made in order to decide whether the traditional average production function (OLS) best fits the data set as compared to the stochastic frontier model selected for this study. The result showed that the SFPF was an adequate representation of the data.
The third null hypothesis is explored that farm-level technical inefficiencies are not affected by the farm and farmer-specific variables, and/or socio-economic variables included in the inefficiency model. The result indicated that the null hypothesis is rejected in favor of the alternative hypothesis that explanatory variables associated with the inefficiency effect model are simultaneously not equal to zero. Hence, these variables simultaneously explain the difference in efficiency among sampled farmers (Table 5).
Table 4: Summary statistics of variables used to estimate milk production and cost function
Variable | Unit | Mean | Std.Dev. | Minimum | Maximum |
Milk output per lactation | Liter | 4989. 03 | 5161. 66 | 300 | 48000 |
Lactation cow | Numb er | 2.94 | 2.03 | 1 | 9 |
Labor | (MD) | 717.4 5 | 410.7 6 | 54 | 3078 |
Green forage | Beli | 202.3 0 | 1243. 97 | 2 | 24300 |
Crop residue | Beli | 38.20 | 59.74 | 1 | 560 |
Cost of lactation cow | Birr | 5611 2.5 | 6339 4.22 | 8000 | 320000 |
Cost of labor | Birr | 2152 3 | 1232 2.82 | 1620 | 92340 |
Cost of green forage | Birr | 1867 6.63 | 1064 05.1 | 160 | 2065500 |
Cost of crop residue | Birr | 3152. 56 | 3775. 36 | 97.5 | 42000 |
Source: Own computation (2020)
Parameter estimates of the SFPF model and cost function
The maximum likelihood estimate of the parameters of the SFPF for milk producers in the North Shewa Zone was presented in Table 6. The results of the model showed the input elasticity for each input in the SFPF. Among four input variables analyzed in the stochastic frontier model, the parameter for lactation cow and crop residue were found to be significant at 10%, as hypothesized as well as green forage was found to be significant at 5%. The parameter estimate for labor turned out to be insignificant. The insignificance of the estimated coefficients for labor implies that the use of this input has no significant effect on milk production in the study area.
Table 5: Generalized likelihood ratio tests of hypothesis for the parameters of the SFPF
Null hypothesis | Df | LR | χ2 value at 5% | Decision |
H0 = βzj = 0 | 10 | 15.46 | 18.31 | Accept H0 |
H0 = γ = 0 | 1 | 10.04 | 3.84 | Reject H0 |
H0 : δ0 = δ1=δ2=. . δ12 = 0 | 12 | 149.3 8 | 21.03 | Reject H0 |
Source: Own computation (2020)
Table 6: MLE for the parameters of the SFPF
Variables | Parameter | Coef. | Std. Err. |
Intercept | β0 | 7.645 | 0.527 |
Ln lactation cow | β1 | 0.109* | 0.062 |
Ln labor | β2 | 0.101 | 0.074 |
Ln green forage | β3 | 0.062** | 0.084 |
Ln crop residue | β4 | 0.074* | 0.039 |
Variance parameter: | |||
λ = σu/σv | 1.33 | 0.173 | |
Gamma (γ) | 0.64 |
Note: ** and * refers to 5% and 10% significance level, respectively.
Source: Model output (2020)
The SFPF model results reveal that the estimated positive and coefficient of lactation cow (0.109), green forage (0.062), and crop residue (0.074) were found to be significant and positive at 5% (green forage) and10% (lactation cow and crop residue) probability level. This indicated that lactation cows, green forage, and crop residue were the most important determinant inputs of milk production in the study area.
This suggests that a one percent increase in lactation cow for milk production, all things being equal, would lead to an increase of 0.109% in the output of milk production. In the same way, on average a one percent increase in the quantity of green forage and crop residue, milk output would increase by 0.062% and 0.074% respectively.
The diagnostic statistics of the inefficiency component reveal that sigma squared (σ2) was statistically significant at 5% which indicates the goodness of fit, and the correctness of the distributional form assumed for the
composite error term. The ratio of the standard error of U (σu) to the standard error of V (σv), known as lambda (λ), is 1.33. Based on λ, gamma (γ) which measures the effect of technical inefficiency in the variation of observed output can be derived (i.e.γ = λ2/([1+λ2])) (Bravo and Pinheiro, 1997). The estimated value of gamma (γ) was 0.64 which indicates that 64% of the total variation in milk output from the frontier is due to technical inefficiency among sample farmers in the study area and 36% of the variation in output from the frontier is due to random noise or random error (beyond the control of the farmers).
The dual frontier cost function derived analytically from the stochastic production frontier shown in Table 6 is given by:
Efficiency scores and their distribution
The MLE results of the stochastic frontier production functions are estimated for the individual farm level TE, AE, and EE independently for sample smallholder farmers. The model output presented in Table 7 indicates that the mean TE of sample farmers was about 0.580 with a minimum level of 0.156 and the maximum level of 0.842.
This means that if the average farmer in the sample was to achieve the technical efficiency level of its most efficient counterpart, then the average farmer could realize 31.12% derived from (1-0.580/0.842)*100 increased milk output by improving TE with existing inputs and technology, using the resource at their disposal in an efficient manner without introducing other improved or external inputs and practice.
In addition, Table 7 shows that the average AE of the sample farmers was about 0.776 with a minimum of 0.299 and a maximum of 0.979. This shows that farmers are not allocatively efficient in producing milk. Hence, a farmer with an average level of AE would enjoy a cost saving of about 20.74% derived from (1 – 0.776/0.979)*100 to attain the level of the most efficient farmer.
Similarly, the mean EE of the sample farmers was 0.447 implying that there was a significant level of inefficiency in the production process. That is the producer with an average EE level could reduce the current average cost of production by 44.81% which is derived from (1-0.447/0.810)*100 to achieve the potential minimum cost level without reducing output levels. It can be inferred that if farmers in the study area were to achieve 100% EE, they would experience substantial production cost savings of 44.81%. This low average level of EE was the total effect of both technical and allocative inefficiencies.
Table 7: Estimated TE, AE and EE scores
Types ofefficiency | Mean | Std.Dev. | Min | Max |
TE | 0.580 | 0.141 | 0.156 | 0.842 |
AE | 0.776 | 0.148 | 0.299 | 0.979 |
EE | 0.447 | 0.133 | 0.102 | 0.810 |
Source: Model output (2020)
The distribution of the TE scores showed that about 47% of the sample households had TE scores of 0.6 to 0.799. 11% of the households’ TE scores fell in the range of 0.2- 0.399. On average, households in this cluster have room to enhance their milk production at least by 42%. Out of the sample households, only 2% had a TE score of greater than 0.8.
This implies that about 98% of the households can increase their production at least by 20%. The AE distribution scores indicated that about 59.25% of milk producers operated above 0.8 efficiency level. The distribution of EE scores also implies that 51.75% of the household heads have an EE score of 0.4-0.599. This also indicates the existence of substantial economic inefficiency than technical and allocative inefficiency in the production of milk during the study period in the study area (Table 8).
Table 8: Distribution of TE, AE, and EE
Efficiency range | TE | AE | EE | |||
Frequency% | Frequency% | Frequency% | ||||
3 | 0.75 | 0 | 0 | 15 | 3.75 | |
0.2-0.399 | 44 | 11 | 1 | 0.25 | 124 | 31 |
0.4-0.599 | 157 | 39.25 | 77 | 19.25 | 207 | 51.75 |
0.6-0.799 | 188 | 47 | 85 | 21.25 | 53 | 13.25 |
0.8-0.999 | 8 | 2 | 237 | 59.25 | 1 | 0.25 |
Source: Model output (2020)
Yield gap due to technical inefficiency
Yield gap analysis is an essential tool to measure to what extent the production could be increased if all factors are controlled. Using the actual output values of the predicted TE indices, the potential output was estimated for each household in milk production per cow per day. Hence, the mean level of the actual and potential milk yield per cow per day was 10.1 liter /cow/day and 19.7 liter /cow/day, respectively. Using the t-test method, the mean difference of the actual and the potential yield was found to be statistically significant at a 1% level of significance. Therefore, the average milk yield gap that is lost due to technical inefficiency, which was the mean
difference between the actual (10.1 liter/cow/day) and the potential output (19.7lit/cow/day) was, 9.6lt/cow/day (Figure 1). This indicates that there is room to boost milk production on average by 9.6 liter/cow/day with the existing level of input use. On average, the money value of milk output that was lost due to technical inefficiency (yield gap) was 153.6birr/cow/day, since the value of 1lt of milk is 16 Ethiopian birr.
Figure 1: Distribution of actual and potential level of milk output
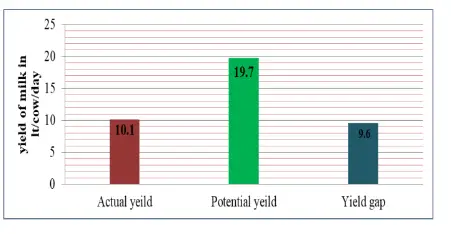
Source: Own computation (2020)
Determinants of inefficiencies
The result of two- limit Tobit model (Table 9) for each significant variable and its marginal effects of change in explanatory variables (Table 10) on TE, AE, and EE were discussed as follows.
Educational
The findings of the study show that education affected TE and EE of milk producers significantly and positively at 1% significance level. The positive sign implies that more educated farmers tend to be more efficient in milk production than the less educated in the study area.
This is due to the fact thatbetter-educatedd household heads can use dairy technology easily and are able to apply technical skills imparted to them. Aone-yearr increase in the educational level of the household head increases the probability of a farmer being technically efficient and economically efficient by 0.34% and 0.01%, and the mean values of technical and economic efficiencies by about 0.92% and 0.97% with an overall increase in the probability and levels of technical and economic efficiencies by 1%, and 0.98%, respectively. The result agreed with the finding of Al-Sharafat (2013).
Total land
The result indicated that total land was a positive and significant effect on AE and EE at a 1% level of significance as expected. This implies that, total land is an important factor in influencing the level of AE and EE in the production of milk or positively contributes to AE and EE of milk production in the study area.
This implies that households who have more land were relatively better in AE and EE. A unit increase in total land (ha) would increase the probability of the farmer being AE and EE by about 1.09% and 0.01% and the expected values of AE and EE by about 0.94% and 0.86% with an overall increase in the probability and levels of AE and EE by 1.13% and 0.87%, respectively.
Dairy experience
Experience significantly and positively affected AE of sampled households at 10% level of significance, which is in line with the hypothesis made. The possible reason is that having more experience and knowledge of on dairy production methods, would increase the probability of the farmers to participating in dairy production. The more dairy production experience, the higher the likelihood of accumulating physical and social capital.
The accumulation of physical and social capital can offer farmers’ better exposure and capacity to produce more dairy production. The study result revealed that, a one year increase of experience in dairy farming would increase the mean values of AE by about 0.04% with an overall increase in the probability and the level of AE by about 0.04%. The finding of this study agrees with the earlier research finding of Al-Sharafat (2013).
Dairy membership
It was found to have a significant and positive effect on AE 10% significance level. The result indicates that the sample farmers who participated in dairy members were more efficient than others. This is because farmers who participate in dairy cooperatives can get different knowledge, information, training, and market access. Moreover, the computed marginal effect result also shows that, a change in the dummy variable, dairy member from (0 to 1), would increase the probability of the farmer being allocatively efficient by about 4.35% and the expected values AE by about 3.22% with an overall increase in the probability and levels of AE by 3.92%.
Amount of concentrate used
The result revealed that, the amount of concentrate feed used by sampled households affected TE positively and significantly at 1% and affect AE negatively and significantly at 5%. This may be due to the fact that concentrated feed provide different nutrients for milking cows which increase the productivity of lactation cow. But the price of this feed is become increasing due to this, farmers may fail to allocate (minimize) the cost of this feed. Furthermore, the computed marginal effect result shows that, a unit increase in concentrate (qt) would increase the probability of TE and decrease the
probability of AE by 0.01% and 0.01% and increase mean values of TE and decrease the mean values of AE by 0.02% and 0.01% with an overall increase in the probability and the level of TE and decrease an overall AE by about 0.02% and 0.02% respectively. This is in line with the research results of Amlaku et al. (2013).
Grazing land
Grazing land significantly and positively affected both TE and EE of the sampled households’ at1% level of significance, which is in line with the hypothesis made. The possible reason is that having more grazing land provides more feed for the milking cows which results increase in milk output. It is the main resource needed by the farmers to feed their livestock which is the main source of feed by providing different fodder and grasses. A unit increase of grazing land would increase the probability of a farmer being both technically and economically efficient by 1.97 % and 0.04% and the mean values of TE and EE by about 5.58% and 3.92% with an overall increase in the probability and the level of TE and EE by about 5.85% and 3.96% respectively.
Type of breed
The result indicated that type of breed was a positive and significant effect on TE at 5% and AE and EE at 1% level of significance respectively as expected. This implies that, cross breed is an important factor in influencing the level of TE, AE and EE in the production of milk or positively contributes to TE, AE and EE of milk production in the study area. Breeds are believed to be genetically improved which makes them more efficient than local breeds.
A change from local to cross breed milking cows increases the probability of a farmer being TE, AE, and EE by 0.85%, 8.69% and 0.07% and the mean values of technical, allocative and economic efficiencies by about 2.33% ,7.54% and 7.61% with an overall increase in the probability and levels of technical, allocative and economic efficiencies by 7.53% , 9.02%, and 7.69 %, respectively. The result is in line with previous studies by Mekdes (2017).
Frequency of extension contact
The result showed that the variable had positive sign and significant effect on TE and EE at 1% level as expected. The reason is that farmers who had more frequency of extension; could lead them to improvements in resource allocation, facilitates practical use of modern techniques and use inputs in appropriate way during dairy production.
A one times increase in frequency of extension of household head increases the probability of a farmer being technically efficient by 0.17% and the mean values of technical and economic efficiencies by about 0.46% and 0.42% with an overall increase in the probability and levels of technical and economic efficiencies by 0.5% and 0.42%, respectively. The finding is in line with the study of Fita et al. (2013).
Table 9: A two-limit Tobit regression results of determinants of TE, AE and EE
Variables | Parameters | TE | AE | EE | |||
Coef. | Std. Err. | Coef. | Std. Err. | Coef. | Std. Err. | ||
Const | δ0 | 0.4261*** | 0.0479 | 0.4517*** | 0.0430 | 0.4517*** | 0.0430 |
Sex | δ1 | 0.0272 | 0.0203 | 0.0029 | 0.0215 | 0.0221 | 0.0178 |
Education | δ2 | 0.0102*** | 0.0017 | 0.0026 | 0.0018 | 0.0098*** | 0.0015 |
Total land | δ3 | 0.0008 | 0.0038 | 0.0120*** | 0.0040 | 0.0087*** | 0.0033 |
Experience | δ4 | -0.0003 | 0.0006 | 0.0012* | 0.0006 | 0.0004 | 0.0005 |
Membership | δ5 | -0.0246 | 0.0150 | 0.0422* | 0.0159 | 0.0061 | 0.0131 |
Concentrate | δ6 | 0.0002*** | 0.0001 | -0.0002** | 0.0001 | 0.0001 | 0.0001 |
Grazing land | δ7 | 0.0595*** | 0.0139 | -0.0129 | 0.0147 | 0.0397*** | 0.0122 |
Type of breed | δ8 | 0.0257** | 0.0118 | 0.0960*** | 0.0125 | 0.0770*** | 0.0103 |
House system | δ9 | -0.0051 | 0.0091 | 0.0094 | 0.0096 | 0.0010 | 0.0080 |
Type of feeding | δ10 | -0.0052 | 0.0153 | 0.0248 | 0.0162 | 0.0130 | 0.0134 |
Extension | δ11 | 0.0051*** | 0.0080 | 0.0005 | 0.0017 | 0.0042*** | 0.0014 |
Distance | δ12 | -0.0002 | 0.0031 | 0.0000 | 0.0022 | -0.0002 | 0.0002 |
Note: ***, ** and *sign represents significance at 1%, 5%, and 10% levels, respectively.
Source: Model output (2020)
Table 10: Marginal effects of change in explanatory variables
Variables | Marginal effect of | Marginal effect of | Marginal effect of | ||||||
TE | AE | EE | |||||||
∂E(y) | ∂E(y ∗ ) | ∂[φ(ZU) − φ(ZL)] | ∂E(y) | ∂E(y ∗ ) | ∂[φ(ZU) − φ(ZL)] | ∂E(y)) | ∂E(y ∗ ) | ∂[φ(ZU) − φ(ZL)] | |
Sex | 0.0268 | 0.0249 | 0.0074 | 0.0027 | 0.0022 | 0.0025 | 0.0220 | 0.0218 | 0.0004 |
Education | 0.0100 | 0.0092 | 0.0034 | 0.0025 | 0.0021 | 0.0024 | 0.0098 | 0.0097 | 0.0001 |
Total land | 0.0007 | 0.0007 | 0.0002 | 0.0113 | 0.0094 | 0.0109 | 0.0087 | 0.0086 | 0.0001 |
Experience | -0.0003 | -0.0002 | -0.0001 | 0.0011 | 0.0009 | 0.0011 | 0.0004 | 0.0004 | 0.0000 |
Membership | -0.0242 | -0.0224 | -0.0073 | 0.0392 | 0.0322 | 0.0435 | 0.0061 | 0.0060 | 0.0000 |
Concentrate | 0.0002 | 0.0002 | 0.0001 | -0.0002 | -0.0001 | -0.0002 | 0.0001 | 0.0001 | 0.0000 |
Grazing land | 0.0585 | 0.0538 | 0.0197 | -0.0121 | -0.0101 | -0.0117 | 0.0396 | 0.0392 | 0.0004 |
Type of breed | 0.0253 | 0.0233 | 0.0085 | 0.0902 | 0.0754 | 0.0869 | 0.0769 | 0.0761 | 0.0007 |
House system | -0.0050 | -0.0046 | -0.0017 | 0.0089 | 0.0074 | 0.0085 | 0.0010 | 0.0010 | 0.0000 |
Type of feed | -0.0051 | -0.0046 | -0.0017 | 0.0234 | 0.0197 | 0.0208 | 0.0130 | 0.0129 | 0.0002 |
Extension | 0.0050 | 0.0046 | 0.0017 | 0.0005 | 0.0004 | 0.0005 | 0.0042 | 0.0042 | 0.0000 |
Distance | -0.0002 | -0.0002 | -0.0001 | 0.0001 | 0.0001 | 0.0001 | -0.0002 | -0.0002 | -0.0000 |
Note: ∂E(y)/(∂X j ) (total change), ∂E(y^* )/(∂X j )(expected change) and (∂[φ(Z U )-φ(Z L)])/(∂X j ) (change in probability). Source: Model result (2020).
CONCLUSION AND RECOMMENDATION Conclusion
The study estimated efficiencies using the stochastic production frontier model. The findings indicated that number of lactation cows, green forage and crop residue were significant determinants of production level. The study also found that farmers can increase milk production by 42% without increasing inputs if they were technically efficient, reduce current cost of inputs by 22.4% with cost minimization way and improve EE by 55.3% when resources are used efficiently.
The positive and significant variables namely; education, total land, dairy experience, dairy membership, amount of concentrate feed, type of breed and frequency of extension in the present study imply that they play great role in enhancing efficiency and productivity of milking cow.
An important conclusion coming from the analysis is that, milk producers in the study area are not operating at full TE, AE and EE level which implies that there is an opportunity for milk producers to increase output at existing levels of inputs and minimize cost without compromising yield with present technologies.
Recommendations
The result of the study provides information and got some policy recommendations to policymakers and extension workers as follows:
Regional government should have a responsibility to keep on the provision of education, and adequate extension services in this area so that farmers can use the available inputs more efficiently under the existing technology.
- Livestock office should give great attention to a cross variety of cows by using artificial insemination in the study area.
- Dairy cooperative should be encouraged by the concerned body like woreda, zonal and regional government.
- The study revealed that the number of lactating cows, green forage and crop residue were found to be highly significant hinting that these are the most critical input to increase milk production and productivity. So that producers and policy makers should use this opportunity to alleviate the existing level of food deficiency & poverty that is to say in designing development policy specifically for improving milk production.
- Adequate and proper management of grazing land should be done by the farmers and concerned bodies.
Conflict of interest
All authors declare that there is no any conflict of interest regarding publication of this manuscript.
Acknowledgment
First and foremost, we would like to thank the Almighty God who gave us the courage through his endless love and blessings that helped us in finalizing the research. We also express our heartfelt appreciation to Salale University for funding this research.
REFERENCES
Africa census. Livestock census statistics on the African continent, 2020.
Aigner, D.J.; Lovell, K.CA,; Schmidt, P.S. Formulation and Estimation of Stochastic Models,2013 Journal of Econometrics, 6 (1), 21-37.
Ali, A.A.; Imad, E.E. ;Yousif, A.K. (Economic Efficiency of Wheat and Faba Bean Production for Small Scale Farmers in Northern State, Sudan, 2012. The Journal of Animal & Plant Sciences, 22 (1), 215-223.
Al-sharafat, A.J. Technical Efficiency of Dairy Farms: A Stochastic Frontier Application on Dairy Farms in Jordan, 2013. Journal of Agricultural Science, 5(3):45-53.
Amlaku, A.S.; Johann, ; Maria,W. Innovation and technical efficiency in the smallholder dairy production system in Ethiopia, 2013. Journal of Agricultural Science and Technology, 3 (2013):151- 164.
Arega, D.;Rashid, M.H. The Efficiency of Traditional and Hybrid Maize Production in Eastern Ethiopia: An Extended Efficiency Decomposition Approach, 2005. Journal of African Economics, 15 (1), 91-116.
Bravo-ureta, B.E.; Pinheiro, A. E. Technical, Economic, and Allocative Efficiency in Peasant Farming: Evidence from the Dominican Republic,1997. The Developing Economies, 3 (1), 48–67.
Central Statistical Agency (CSA). Federal Democratic Republic of Ethiopia Central Statistical Agency Agricultural Sample survey 2020/21. Volume Ii Report On livestock and Livestock Characteristics (Private Peasant Holdings), Addis Ababa.
Coelli, T.J. Recent Developments in Frontier Modeling and Efficiency Measurement, 1995. Australian Journal of Agricultural Economics, 39 (3), 219-245.
Coelli, T.J.; Rao, D.SP.; Battese, G.E. An Introduction to Efficiency and Productivity Analysis. Kluwer Academic Publishers, Boston, Dordrecht/London, 1998. Pp: 134-249.
Fita, L. Trivedi,M.M. ;Patel,A.M. ;Tassew,B. ; Joshi,C.G. Determinants of Technical Efficiency of the Dairy Farmers in Ada’a District of Oromia State, Ethiopia,2013.Iranian Journal of Applied Animal Science. 59-65.
Greene, W.H. Econometric Analysis, 5th Ed. Prentice Hall, Upper Saddle River, Newjersey, 2003.
Ittersum, M.K.; Cassman, K.G.; Grassini, P.; Wolf, J. ; Tittonell, P. ;Hochman, Z. Yield Gap Analysis with Local to Global Relevance Review. Field Crops Research, 2013, 143, 4-17.
Meeusen ; Van Den Broeck, J. Efficiency Estimation from Cobb-Douglas ProductionFunctions with Composed Error, International Economic Review, 1977, 18 (1), 435-444.
Mekdes, G. Technical Effieiency of Commercial Dairy Farms; the Case of Sululta District, Oromia Regional State of Ethiopia, 2017.
Nathaniel, M. ;Raphael, M. ;Tsehay, R. Akke van der, Z. Jan van der, L. White Gold: Opportunities for Dairy Sector Development Collaboration in East Africa Centre for Development Innovation. Wageningen UR, 2014.
Nega, W.; Simeon, E, Technical efficiency of smallholder dairy farmers in the Central Ethiopian highlands. Pp. 12-18 in Proc. International Association of Agricultural Economists Conference, Gold Coast, Australia, 2006.
Sharma, K.R. Leung, P.; Zaleski, H.M. Technical, Allocative and Economic Efficiencies in Swine Production in Hawaii: A Comparison of Parametric and Nonparametric Approaches, 1999. Agricultural Economics, 20 (1), 23-35.
Yamane, T. Statistics: An Introductory Analysis, 2nd Edition, New York, 1967.